One important part of the MeMAD project is working with potential users of the tools developed, as well as presenting the research and development work to professional communities and wider public audiences. In November and early December of 2019, we carried out user evaluation with professional translators to find out what they thought about using machine-translated subtitles as part of their workflow and how it would affect their productivity. This kind of user evaluation represents the evaluation phase of the user-centred design approach that is central to the development work in the MeMAD project. The evaluations were organised by the project partners at University of Helsinki and Finnish Broadcasting Company Yle, and a total of 12 professionals working with audiovisual translation participated in the experiments. So what happened in the tests and what did the professionals think?
Pilot testing machine translation and post-editing for subtitles
In our user evaluation experiments, the translators worked on creating subtitles for short clips of different types of videos – from European election debates to youth-oriented talk shows – in four different language pairs: English-Finnish, Finnish-English, Finnish-Swedish and Swedish-Finnish. We collected data about both the process of “post-editing” or correcting machine translated subtitles and creating subtitles “from scratch”, without using machine translation. The collected keylogging data allows us to compare the time it took to complete the tasks and the number of keystrokes used by the translators. Measuring the number of keystrokes allows us to determine the amount of technical effort the translators used to complete different types of tasks. Analysing such processes involves also another type of effort, namely the cognitive effort required to come up with a translation solution, to spot potential errors and decide on the necessary corrections. This type of effort is more difficult to capture, although some information can be gained from analysing pauses in the keylogging data. Alongside process data, we also collected feedback from the translators both as numerical assessments of perceived effort and various other aspects of the user experience and through interviews.
What we observed in the process data was that, on average, post-editing machine-translated subtitles was faster than creating subtitles from scratch, and also involved fewer keystrokes, as is shown in the figures below.
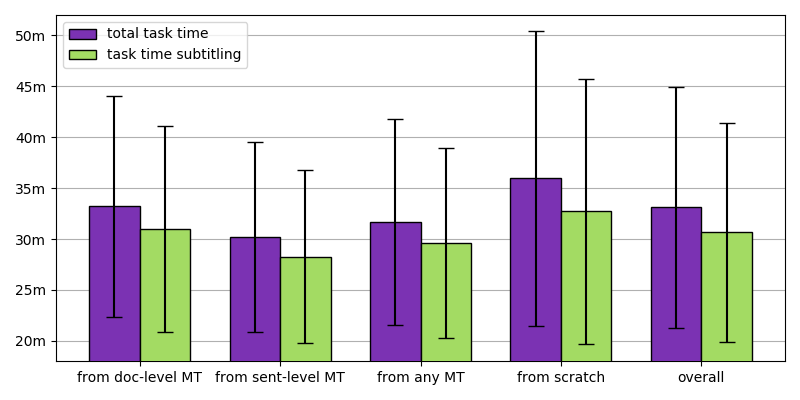
Comparison of task times with and without machine-translated subtitles. Total task time includes time spent on subtitling as well as time spent on terminology searches and other research
The types of keystroke used also showed some differences in how post-editing differs from translation from scratch. Because the machine translation output already contains some of the words that will end up in the final translation, the translators needed fewer keystrokes to produce text. On the other hand, correcting the output involves deleting incorrect or unnecessary words, increasing the number of keystrokes needed for this purpose. In subtitle translation, keystrokes are also needed for creating or changing the subtitle frames where the text is shown, and their timing on screen.
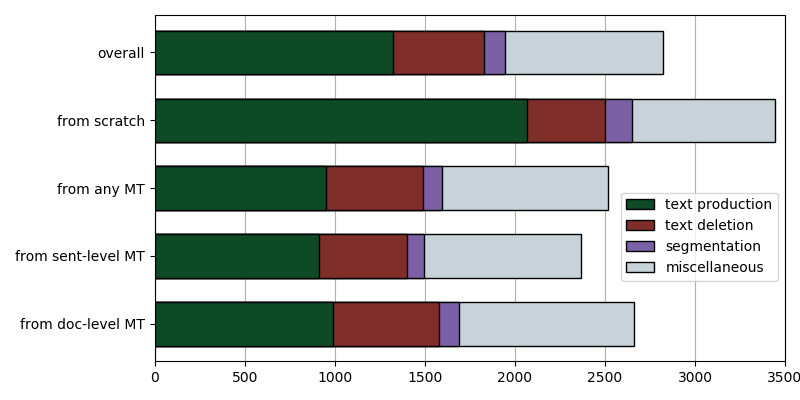
Average number and types of keystrokes when creating translated subtitles from scratch and when post-editing machine translated subtitles
In these experiments, we used two different types of output: one produced with a sentence-level machine translation model and the other with a document-level model. On average, the translators were a bit faster when working with output from the sentence-level model, but no clear difference could be seen between the two. What we found instead was that there were noticeable differences in task duration and keystrokes between different translators and different types of clips. A more detailed analysis of these differences is still ongoing, but at first glance, they suggest that the machine translation output was more helpful with the EU debates, for example, than with the more colloquial style of the youth programme. Training data for the machine translation systems included substantial amounts of text dealing with EU topics, which may explain better output. On the other hand, informal speech is a challenge for machine translation, because suitable training data is more difficult to find.
So what did the professional translators think?
Learning about the experience of real users interacting with the tools and outputs is an important part of the development of the MeMAD prototypes, and for this reason we collected feedback from the translators participating in the experiment. Immediately after the subtitling tasks, the translators filled out a questionnaire based on the User Experience Questionnaire. This questionnaire is widely used to collect users’ impressions of usability and efficiency of a tool. It asks the user to evaluate various aspects of their experience according to positive vs negative adjective pairs. Responses from the translators participating in the experiment are shown in the image as an average for each of the four language pairs. The value 0 represents a neutral assessment, with longer bars to the positive or negative side indicating stronger reactions.
Interestingly, the translators participating in our tests appeared to have somewhat different experiences depending their language pairs. On average, evaluations by the translators working with English-Finnish or Swedish-Finnish appear to be more negative than in the two language pairs where Finnish was the source language. Particularly the translators who worked with the Finnish-English machine translation output characterised their post-editing experience in slightly positive terms as easy, relaxed, efficient as well as exciting and motivating. On the whole, the translators did not consider post-editing particularly difficult or complicated, but found that it could be somewhat annoying and that using the machine-translated output limited their creativity. Their evaluation of the segmentation and timing of the subtitle frames was quite negative, and this is an area where more work is needed in developing our system.
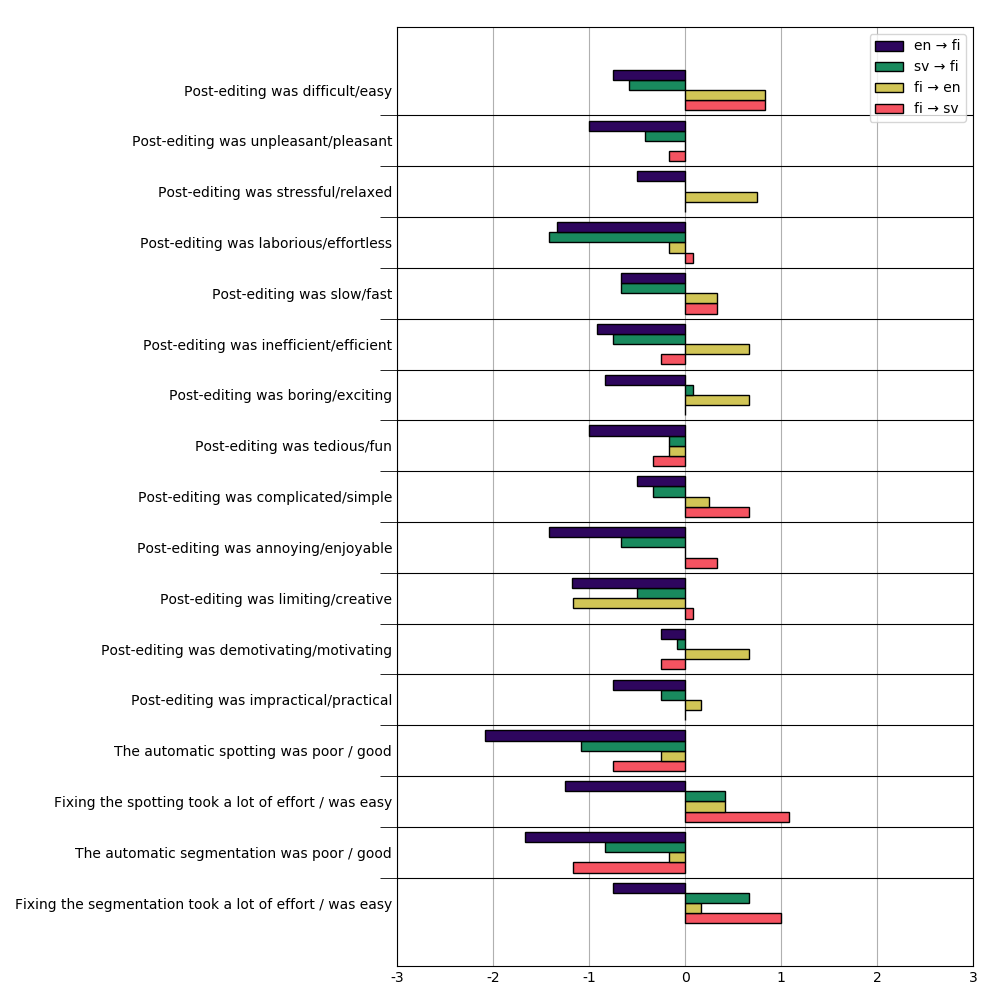
User Experience Questionnaire scores for different language pairs
In addition to the questionnaire, we conducted short interviews with the translators to hear about their experience in more detail. The translators had both positive and negative things to say about machine translation quality and the experience of post-editing machine translated subtitles. Nearly all commented positively on the overall quality of the machine translation, with several participants noting it was clearly better than they expected based on what they have previously seen from other machine translation systems. In particular, the translators commented that they often found the terminology available in the output helpful, even if the precise wording required some editing.
A major issue with the output appears to have been the segmenting and timing of the subtitle frames. All of the translators commented negatively on issues such as translations sometimes being out of sync with the audio and sentences being incorrectly split into two or more segments. Problems identified in the machine translation outputs included missing words, mistranslated or “odd” words and unidiomatic structures. Two of the translators also specifically mentioned that the machine translation was using too many words – a beginner’s mistake, one of them called it. This demonstrates something that is very typical of audiovisual subtitle translation: subtitles are limited by both space restrictions and the time each subtitle frame can appear on the screen. For this reason, subtitle translators need to find ways to condense the written translation of a spoken message into fewer, more economical words. This type of condensation appears to be something that is not yet reproducible with the current models. Work on optimising the models with more subtitle translation data is currently ongoing.
Of the 12 translators, 10 said they could see themselves using machine translation and post-editing for subtitling, although they would like to see some improvements in the quality of the machine translation and subtitle segmentation. Half of the participants also noted that they would not use machine translation for all content, rather, it would depend on the type of programme and subject matter. Some saw it as most useful for content they were not very familiar with to help with terminology. Others thought it would be better to use machine translation only if they knew the subject matter well enough to be sure they would spot possible errors.
How to improve machine translation for subtitling?
The translators who participated in the post-editing experiments had a number of suggestions on how machine translation for subtitling could be improved:
- The most common development need brought up by the translators was improving the segmentation and timing of the subtitles.
- Some suggested showing the machine translation as a whole, perhaps accompanied by a script of speech recognition output of the original audio.
- For machine translation quality, the translators mentioned issues like more condensed language, better cohesion, and adapting to the genre of the programme, for example, more formal or more colloquial style of speech.
- Some of the translators also wished the system worked more like the translation memory tools commonly used in translating technical documents. They thought it would be useful if either individual translators or groups of translators translating a television series, for example, could add their own material and see how things were translated previously.
- One of the participants also wondered whether it would be possible for the machine translation system to use the visual information in the video, like a translator does. This is something that we in the MeMAD project are in fact investigating!
Overall, the experiments with professional translators provided us with much valuable information from the perspective of potential users. This point of view will be used to further inform our development work during this final year of the project.
Presenting automated subtitle translation to the professional communities and the public
At the end of January 2020, the MeMAD work on machine translation of audiovisual subtitles also got the spotlight in the Translating Europe Workshop “Machine translation and the Human Translator” in Tampere, Finland. This one-day event organised by Tampere University in collaboration with the European Commission Directorate General for Translation, the European Master’s in Translation network and University of Turku brought together some 100 participants – professional translators, translation scholars and students. The talks given during the event were also streamed online with simultaneous interpreting. This event gave us an opportunity to discuss the work we have done on machine translation models for subtitle translation. We also gave the participants a first peek at the results of our user evaluation experiment.
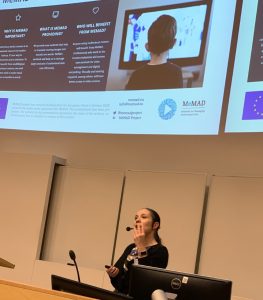
Maarit Koponen from University of Helsinki presenting the MeMAD project at the “Translating Europe” Workshop in January 2020 (Photo credit: Juha Eskelinen)
The MeMAD work on multilingual and multimodal machine translation also got visibility through an interview where postdoctoral researcher Maarit Koponen talks about the possibilities and challenges of machine translation in general, and the goals of the MeMAD project in particular. The interview, published by the communications department of the University of Helsinki in December 2019, was shared and discussed actively in social media like Twitter and Facebook groups aimed at professionals and researchers in the translation field.