At the end of the second year of the MeMAD project, different aspects of the prototype were chosen for evaluation, based on product maturity. There were four tracks of evaluation: video editing assistance, searching, intralingual subtitling with the help of automatic speech recognition (ASR) and interlingual subtitling with the help of machine translation (MT). This post provides the news from the first two tracks. You can read about subtitling with the help of MT here.
In all evaluations, participants filled out User Experience Questionnaire type forms (UEQ) after each task, adapted to focus on the task itself rather than the user interface. After each evaluation session there was a brief semi-structured interview. Additionally, in the video editing assistance and searching evaluations think-aloud data was collected.
Automatically generated metadata, particularly ASR transcripts and MT, was found to be useful in both video editing assistance and in archive searching, though there is still room for improvement.
Editing assistance
For the editing assistance case, three in-house video editors at Yle were given the task of making a 2-minute video summary of the lead candidate debate of the 2019 European parliament elections, based on a rough script. An hour and half of video material was enriched with speech recognition transcripts, machine translations for the segments in French, face recognition data for the lead candidates and the hosts, and named entity recognition. This automatically generated metadata was imported into a professional video editing tool as markers.
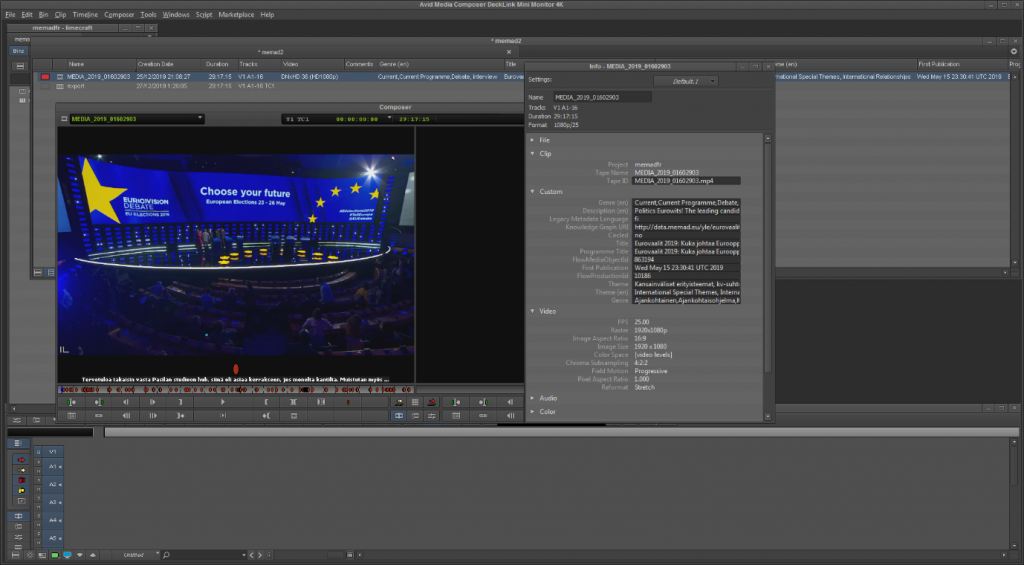
Image 1: Markers shown on the video timeline in the video editing software.
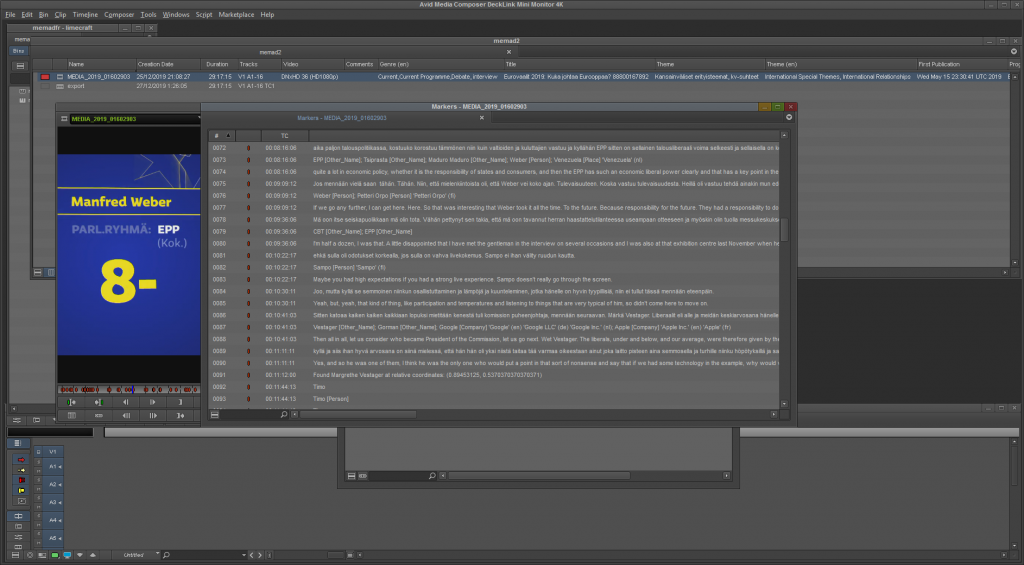
Image 2: The contents of the markers in the video editing software.
User feedback
The amount of metadata in the markers was difficult for the video editing software to handle, which led to some technical difficulties during the test sessions. Despite this, feedback from the test subjects was mainly positive. They found the metadata interesting and helpful, but at the same time they did not find it very relevant for their daily work, as they normally get very detailed scripts from journalists to work with, with exact timecodes marked for the clips needed. In their usual workflow, the ones finding the exact time codes are journalists who write the scripts, not the video editors themselves.
The participants noted in interviews that they did not pay much attention to the quality of the different kinds of metadata; it was enough for them that they found the right segments, and for example word for word accuracy in the transcript was not needed. The test subjects were more critical of the search functionalities: not being able to search for a person (face recognition) and what they said (ASR transcript) at the same time, being limited to “exact match” search (ie. if searching for “immigrants Mediterranean” they would not find the segment where “immigrants in the Mediterranean” was mentioned), and the searched word not being highlighted in the transcript. They were also somewhat critical of the segmentation, finding the segments in the markers too long; the participants would have preferred shorter, perhaps sentence-long segments. Longer segments meant that even when they found what they were looking for in the transcript, they still had to browse through a minute or so of video to find the exact place where the search term was used. Segmentation here refers to how the transcript was split up into separate markers. While there were some shorter segments, most were longer, containing up to a minute or so of speech.
None of the participants used the named entity recognition data in their tasks, and one of the three participants did not use the face recognition data either, preferring to look the lead candidates up on Google.
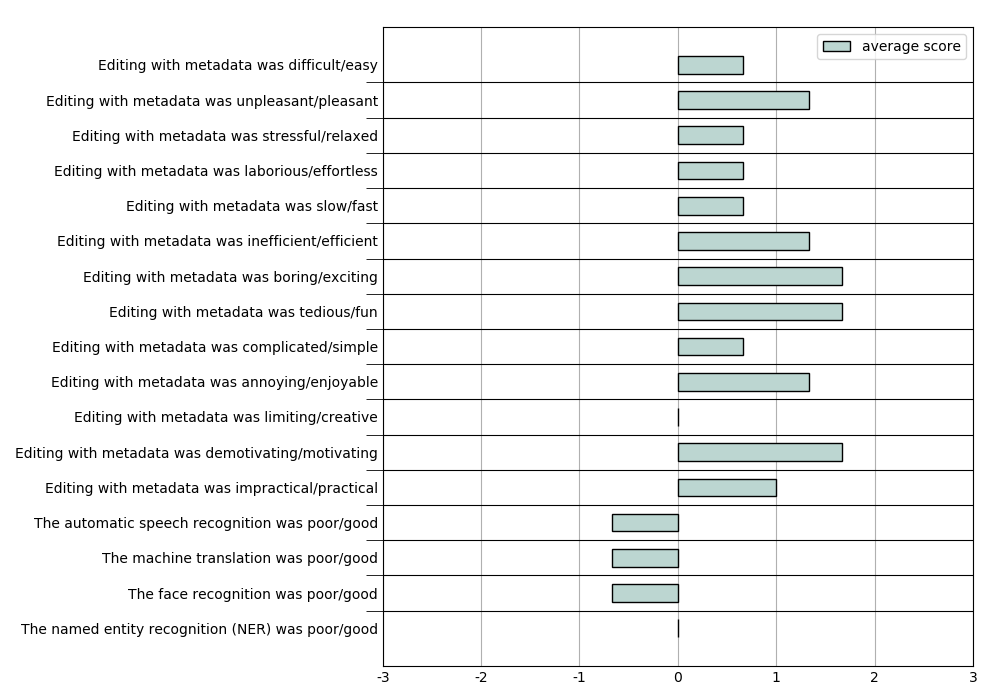
Image 3: Average User Experience Questionnaire (UEQ) scores for video editing assistance.
Future improvements
Professional video editors found the editing assistance useful in the context of the given tasks, but not relevant to their daily work because they typically receive detailed scripts from journalists, ie. the journalists do the work of finding the correct segments, not the video editors themselves. In future evaluations we will focus on how the tools could help journalists who do video editing for their own stories.
There is room for improvement in the presentation and searchability of the metadata as well. It would be beneficial to be able to search for segments where a certain person speaks about a topic, combining face recognition and speech recognition data. Segment lengths also need to be examined, though in longer videos shorter segments may result in too many markers for a video editing tool to handle.
In this evaluation, the search functionalities (searched words not being highlighted within the transcript, exact phrase search) were more the result of the video editing software used than the metadata generation, and as such are outside of the scope of this project.
Searching
In the archive search case, two Yle journalists and three archivists from KAVI (Finnish National Audiovisual Institute) were given a series of search tasks to perform, using a limited library of material (408 television and radio programmes taken from the collections of Yle and INA, 210 hours in total). Languages spoken in the material were mainly Finnish, French, English and Swedish, with small segments of other languages. The search tasks were carried out on Limecraft’s Flow platform.
The test materials were enriched with
- legacy metadata (original, manually produced programme descriptions)
- automatic speech recognition transcripts for the four main languages
- machine translations into English for those transcripts that were in Finnish, French or Swedish
- named entity recognition.
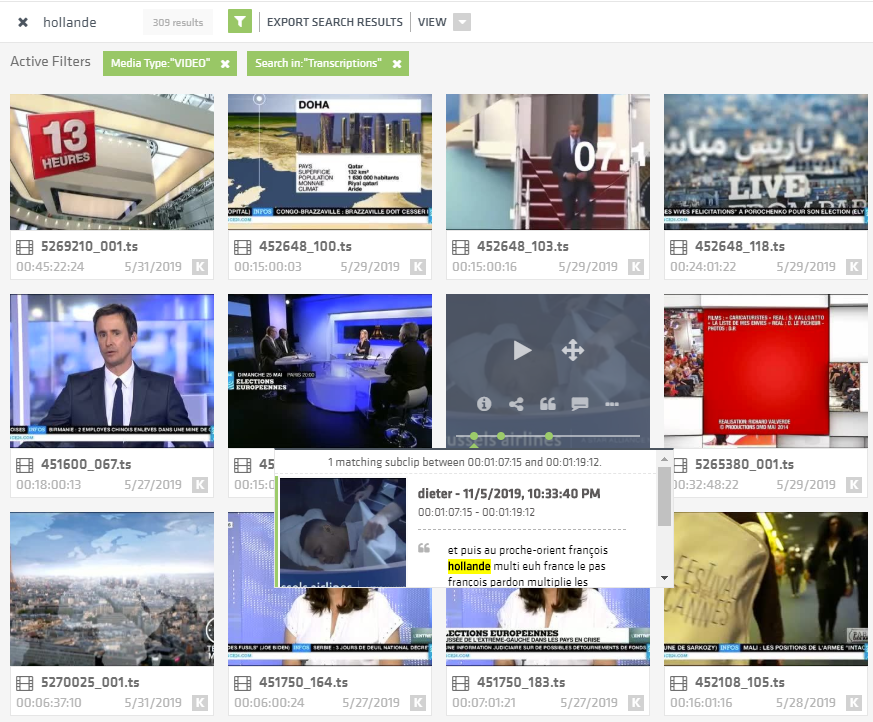
Image 4: The Flow platform library shows search results for the term “hollande”.
Each participant was given six different search tasks, based on real-life search scenarios. They would first attempt to carry out the task with the help of legacy metadata only, and then again with the help of all available metadata. Each search task was representative of a type of search commonly performed by archivists.
The search types were:
- searching for a specific program
- searching for a program type
- searching for a topic
- searching for a specific person speaking about a specific topic
- searching for a location
- searching for an object.
User feedback
The participants were given a short briefing about how Flow worked and what functionalities were available at the start of the evaluation session. They would have benefited from having a bit more time to get accustomed to Flow; as it is, they sometimes forgot entirely about some of Flow’s functions. The logic of Flow’s search function was also a source of some frustration for the participants from KAVI, as they were accustomed to searching by topic or keywords instead of full text descriptions. When searching for people talking about wind power, for example, they might search for “renewable energy”, which did not result in any hits.
The automatically generated metadata did not include visual elements, and it was difficult for the participants to complete the tasks where location and object were searched for. When searching for horses, for example, the participants mostly found horse-related metaphors in the transcripts, and finding actual horse images was more difficult. Finding a specific person speaking about a specific topic proved to be the most difficult in this evaluation; none of the participants completed that task.
Much like in the video editing case, the participants said that they did not pay much attention to the quality of the metadata. This is reflected in the results; Image 2 shows that the quality of the metadata was assessed as 0 or close to 0 (neither good nor poor) for most tasks. Otherwise the UEQ results strongly correlate with whether the participant was able to complete the given task or not; the scores are largely negative when they could not complete the task, and positive when they could. In the interviews some of the participants commented on this, noting that they found the enriched metadata very interesting and useful even if they could not complete all tasks. Most participants said that they would use this kind of automatically generated metadata in their work.
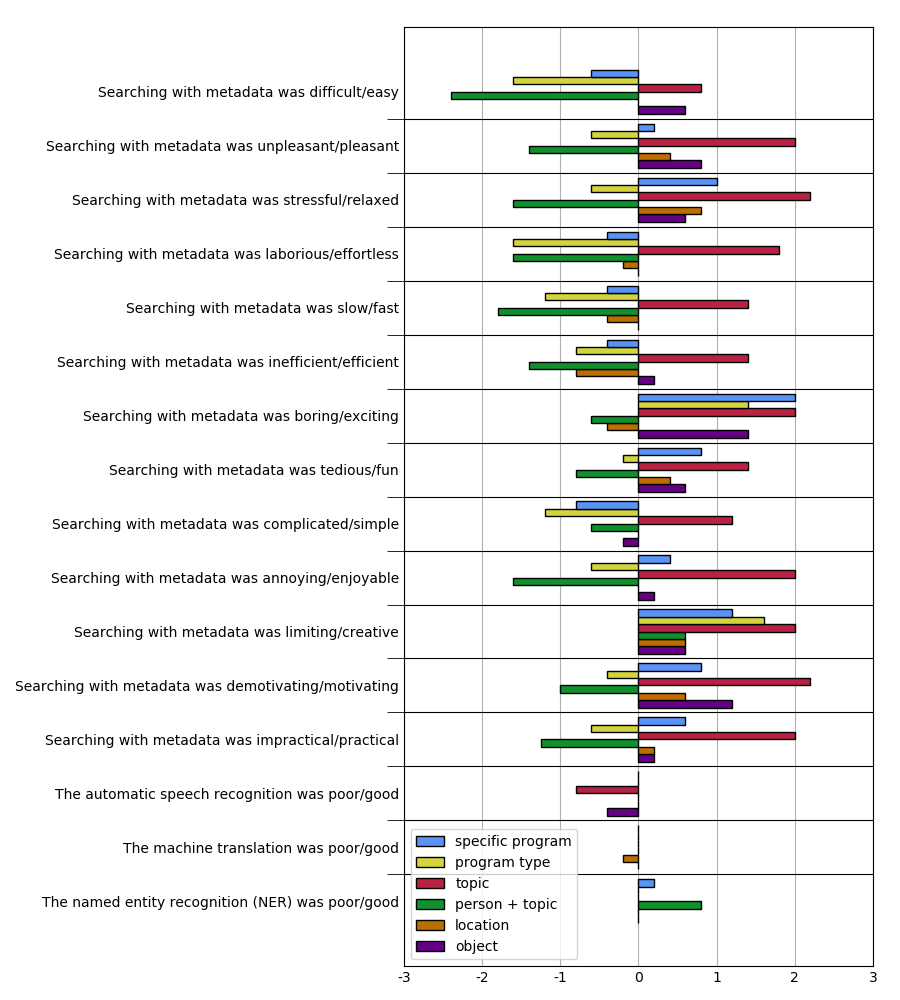
Image 5: Average User Experience Questionnaire (UEQ) scores for searching.
Future improvements
While the participants for this evaluation were at times critical in their UEQ assessments, they were enthusiastic about the potential in these technologies. Their feedback is valuable in developing the MeMAD prototype.
It is clear that visual elements need to be included in the metadata for it to be useful in certain types of search tasks. Future evaluations will incorporate face recognition and visual object detection. The same participants should be used in future evaluations, where possible, since they are already familiar with the platform and can compare results.
What’s next?
Further evaluations are planned in Year 3 for both of these use cases, with improvements to the metadata and the test set-ups. The third and final round of evaluations is scheduled to start in June 2020.