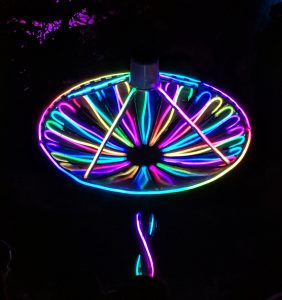
Image by James Sloan https://unsplash.com/@sloandaddyslim
MeMAD project partners have received recognition for their accomplishments. Three project innovations were recognized by the European Commission’s Innovation Radar. Innovation Radar is a European Commission initiative that champions potential innovations and innovators in EU-funded research and innovation framework programmes. The innovations are categorised in four different levels of market maturity.
- Exploring: Innovations are actively exploring value creation opportunities.
- Tech Ready: There is progress on technology development process, e.g. pilots, prototypes, demonstration.
- Business Ready: They are putting concrete market-oriented ideas together, e.g. market studies, business plans, end-user engagement and
- Market Ready: The innovation is outperforming in innovation management and innovation readiness and considered commercially viable.
The key innovators of this innovation are MeMAD partners Aalto University and Lingsoft.
Aalto University has studied new deep learning methods for speech-to-text, speaker diarization and spoken named entity recognition. They have found successful methods to adapt deep neural networks trained on big background data (e.g. text or untranscribed or unrelated speech) with small target domain data. Aalto has also studied end-to-end methods that combine speech, speaker and named entity recognition and even machine translation into single systems which would avoid cascaded errors that often result from the conventional pipeline architectures.
Lingsoft continuously develops the speaker segmentation, speech recognition and Named Entity Recognition. Each of these services are available to Lingsoft customers and partners via an API. In addition, they will be available through the European Language Grid in the future. Lingsoft is committed to developing these methods further to solve real-world language problems. We find solutions that benefit the whole language management process.
Together, their research has been designated at the maturity level Tech Ready
New methods for people and music identification for more accurate video segmentation
The key innovators of this innovation are MeMAD partners University of Surrey and Institut National de l’Audiovisuel (INA).
Surrey’s work has focused on analysing human techniques for identifying recurring characters in moving imagery with a view to generating greater cohesion, and ultimately informing improved computer sequencing across multimodal narrative. They have also been researching the application of story grammar methods to narrative segmentation.
INA’s work has focused on building DNN multimodal analysis tools. The software inaSpeechSegmenter allows to split audio signals into speech, music and noise segments, and to infer speaker gender. It achieved the first position on MIREX 2018 voice activity detection challenge. This software was also highlighted in an earlier MeMAD blog post for describing women and men representation in French TV and radio: https://memad.eu/2019/10/01/analysing-1-million-hours-french-tv-radio-describing-gender-equality/
Together, their research has been designated at the maturity level Exploring
Novel metadata structures to support multimedia content descriptions for searching and browsing
The key contributors to this task are MeMAD partners University of Surrey, EURECOM and Institut National de l’Audiovisuel.
EURECOM is promoting the use of semantic web technologies for developing expressive knowledge graphs representing both legacy audiovisual metadata as well as results of automatic multimedia analysis such as face recognition or named entity disambiguation. EURECOM has adopted and extended the EBU Core ontology model, the flagship metadata model for describing audiovisual resources. EURECOM has designed and developed an exploratory search engine enabling to search and browse large audiovisual archives as well as getting recommendation at the fragments level. This web application makes use of another innovation named SPARQL Transformer enabling to automatically build a RESTful API on top of any knowledge graph.
The University of Surrey’s role has been to apply corpus linguistics, multimodal analysis and narrative storytelling techniques to the understanding of human-derived video descriptions, as a means of informing future developments in computer-assisted video captioning. Our work has taken place at the intersection of face recognition, scene and topic detection and video diarisation and segmentation which has been undertaken by project partners, with the ultimate goal of improving video accessibility and retrieval through the semi-automation of video descriptions.
INA has developed multimodal analysis tools that have been made open-source and that were used by the University of Surrey. inaSpeechSegmenter allows to split audio streams into speech, music and noise excerpts. inaFaceGender performs face detection, face tracking, and face classification into women or men. This information provides a low-level description of multimedia collections and was found to be very robust.
Together, their research has been designated at the maturity level Exploring